Cognitive computing strengthening industry
Usage of Cognitive Computing
Add bookmark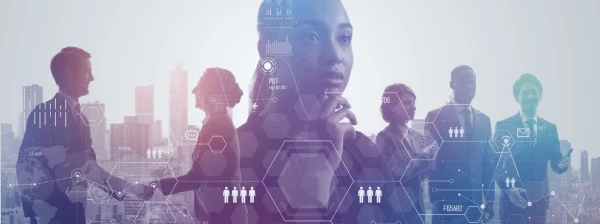
What is Cognitive Computing?
Artificial intelligence and cognitive computing work from the same building blocks, but cognitive computing adds a human element to the results.
AI is a number cruncher, concerned with receiving large amounts of data, learning from the data, finding patterns in that data, and delivering solutions from that data.
Cognitive computing, on the other hand, takes those outputs and examines their nuances.
Also read: Enterprise AI
The cognitive computing branch of AI is able to operate with unstructured data which is any data that is not contained in a formatted record. Unstructured data files often include text and multimedia content. Examples include e-mail messages, word processing documents, videos, photos, audio files, presentations, webpages and many other kinds of business documents.
Note that while these sorts of files may have an internal structure, they are still considered “unstructured” because the data they contain doesn’t fit neatly in a labelled database. Sensor data-both in the form of video and audio transmissions, social media data, and communications data are all forms of unstructured data.
Simply put to computers – the language that we humans speak is in an unstructured format – and the branch of AI that is capable of dealing with ‘our’ form of communications is ‘Cognitive Computing.’
As the majority of the data gathered by enterprises are in an unstructured form, it becomes a necessity to deploy cognitive computing models to take advantage of AI.
After surveying 200+ digital transformation leaders on the matter, we compiled their input into our latest, in-depth report: DX Talent Reimagined. Download now and discover:DX Talent Reimagined: 2022 Industry Report
Download Now
Cognitive computing in businesses
In this section we explore methods or models of cognitive computing.
Internet of Things
Using deep learning, a system can be trained to recognize images and/or sounds-from an unstructured form. The systems learn from labelled examples in order to accurately classify new images or sounds.
For instance, a computer can be trained to identify certain sounds that indicate that a motor is failing. The sound can be detected in the form of audio signals by sensors. These audio signals can then be relayed to AI, or to be more specific- a cognitive computing model, which can then analyse the patterns and identify any irregularities.
This system of detecting signals via sensors and then analysing them through a central ‘AI powered’ computer is known as ‘Internet of Things’.
This kind of application is being used in automobiles and aviation. Such technology is also being employed to classify business photos for online auto sales or for identifying other products. A photo of an object to be sold in an online auction can be automatically labelled, for example. Image recognition is being put to work in medicine to classify mammograms as potentially cancerous and in genomics to understand disease markers.
The following are examples of some enterprises that are making use of cognitive computing and IoT to leverage the massive amounts of unstructured data that they gather:
- Harley Davidson Motor Cycles has transformed one of its York-based factories into an IoT-enabled plant. This shift sent shockwaves through the established system - the 21-day production schedule shrunk to a mere 86 seconds
- Walt Disney World have created ‘Magic Band’, a wearable wristband containing RFID tags. Disney World visitors can check-in to the park, buy food, and gain fast pass on rides by tapping the band on receivers. Disney can then use this data to track the movement of visitors though the park and determine which areas, rides and attractions are the most popular and which areas of the park require more attention.
- HSBC is a big fan of biometric technology for financial services. It reminds people that customers can use Touch ID fingerprint-recognition, voice recognition and Selfie ID, to log-in with a photo.
Natural language processing
Most of the world’s data is unstructured i.e., raw text in English or another human language. As long as computers have been around, programmers have been trying to write programs that understand languages like English. The reason behind this continual interest in NLP is obvious humans have been writing things down for thousands of years and it would be really helpful if a computer could read and understand all that data.
Text analytics—using natural language processing (NLP) or machine learning— is being used to structure unstructured text. For example, organizations can extract entities (people, places, or things), themes, or sentiment from call center notes. That information can then be combined with other information about customers to build predictive models. For example, entities, concepts, and themes can be clustered using statistical techniques.
Additionally, companies can use survey responses verbatim, assigning entities, concepts, and themes as data and using this for prediction without structured data. Some organizations say that these models outperform models that use only traditional structured data.
Natural language processing use cases
The following are examples of some enterprises that are making use of cognitive computing together with NLP to leverage the massive amounts of unstructured data that they gather:
- In 2016, Citibank introduced voice biometrics to verify the identities of customers contacting their call centers. Voice authentication uses biometrics to verify customers’ identities while they’re explaining an issue to a customer service representative over the phone. It analyses unique characteristics in a person’s vocal pattern and cross-checks them against a pre-recorded voice print to verify their identity. Voice authentication cuts out the cumbersome process of verifying a customer’s identity through ID numbers and personal details, reducing friction for customers and allowing representatives to provide meaningful help faster. It takes less than one minute for a customer to set up a voiceprint, and over one million customers in the Asia Pacific region used voice authentication within one year of its release.
- JP Morgan Chase & Co. bank has invested $9.6 billion in cognitive computing, which has already netted a huge payoff. The first fruits of their investment is Contract Intelligence, or COiN, which uses machine learning to interpret documents. COiN reviewed 12,000 commercial credit agreements and provided analysis in a matter of seconds. What makes that remarkable is the same task takes 360,000 attorney hours to complete.
- Spell check is a form of NLP that everyone has used. It’s unobtrusive, easy to use, and can reduce a lot of headaches for both users and agents alike. Not every user is going to take the time to compose a grammatically perfect sentence when contacting a help desk or sales agent. Almost all companies that offer online form fill-ups knows this, so they made sure their contact form was equipped with spell check to make users’ lives easier. This also makes their employees’ lives easier, too. Error-ridden customer messages can be difficult to interpret, leading to miscommunication and frustration for all.
Chatbots in customer experience
Chatbots have been in the market for a number of years, but advancements have led to a better understanding of language and are more interactive. Here, based on who you are (e.g., whether you have status with the company) and what you asked for (using NLP for text analysis), you will be routed to the right customer representative to answer your specific questions.
Companies use chatbots for personalized shopping that involves understanding what you and people similar to you bought, in addition to what you are searching for. Many companies are successfully using customer support chatbots services to streamline some of the work that would traditionally fall to representatives.
Also read: DX Talent
Models built with NLP algorithms are the brains of these chatbots. They’re trained using text data from past conversations between customer support agents and customers. This training allows the bot to identify the meaning of the customer’s requests using context clues and provide an answer that a human will understand.
The whole process can take place almost instantaneously, so the customers never have to wait for an answer. In the meantime, the bot collects new data as it interacts with customers, so it can actually continue to learn from experience just as a human would.
Chatbots in customer experience use cases
Here are a few examples and use cases:
- As a market leader in both mobile banking use and AI implementations in the U.S. – BANK OF AMERICA introduced Erica, (from the word AmErica) to send notifications to customers, provide balance information, suggest how to save money, provide credit report updates, pay bills and help customers with simple transactions.
- Since its introduction in 2018, BofA boasts that 10 million people use Erica, and Erica is at the 100 million marks in completed client requests. There are over 400,000 unique ways a customer can ask Erica a question—and that number is growing daily.
- In May of 2018, buoy announced a partnership with where if medically appropriate, Buoy’s chatbot would connect patients to a nearby Minute Clinic location. Buoy also works independently to listen to medical concerns, help identify what’s wrong, and guide you to the right care, saving both patient and ER time and resources.
- Travel brand hipmunk utilizes a conversational bot that not only helps with booking hotels and airports, but can give you directions, find you deals, and stays in contact with you after you arrive to suggest points of interest. Plus, it’s got a sense of humour, complete with appropriate GIF usage. It’s also easy to access, meeting customers where they already are Facebook, Skype, and even Slack.
Predictive analytics
Predictive analytics refers to using historical data, machine learning, and artificial intelligence to predict what will happen in the future. This historical data is fed into an algorithm that considers key trends and patterns in the data.
As with most cases, the data is usually in an unstructured form and hence most predictive analytics models use cognitive computing. The model is then applied to current data to predict what will happen next. Using the information from predictive analytics can help companies—and business applications—suggest actions that can affect positive operational changes.
Analysts can use predictive analytics to foresee if a change will help them reduce risks, improve operations, and/or increase revenue. At its heart, predictive analytics answers the question, “What is most likely to happen based on my current data, and what can I do to change that outcome?”
Accessing and using unstructured data / predictive analytics use cases
Amazon uses Predictive Marketing to recommends products and services to users based on their past behaviour. Some say that recommendations are responsible for as much as 30 percent of Amazon’s sales.
Customer loyalty— Preventing a wandering eye from switching brands is one thing, but what can brands do to prevent the wandering eye in the first place? AI data analysis across digital channels offers brands the opportunity to deploy strategic marketing efforts that micro target specific customer segments. By knowing what a customer wants when they want it and on what platform they want it on, customers are met where they are with the solutions they’re looking for. When a customer’s needs are met, they have no reason to look for alternatives.
Key questions for enterprise executives
AI and cognitive computing are not a magical solution that applies to every single use case. Often, companies embark on an AI development journey without a clear understanding of the value it should bring to their business. As a result, many data science and machine learning projects don’t have clear KPIs and simply drain R&D budgets.
An executive of an enterprise who’s looking to implement the power of cognitive computing must address a few key questions.
Do I have any data? Do I have enough data?
Yes, this sounds like an obvious question, but machine learning works best when you have significant amounts of data, with no signs of slowing down its accumulation. There may be more cost-effective ways of analysing your data. (Defining a “relatively modest set” is a matter of perspective: Several years ago, “relatively modest” meant several hundred gigabytes. Today, it may mean dozens of terabytes or even several petabytes.)
What shape is my data in?
If your data is coming from a variety of sources, or if it hasn’t been cleaned and standardized into a consistent set, you won’t get value from any analytical exercise. “Clean data is better than big data” is a common phrase among experienced data science professionals. If you have reams of business data from years ago, it may have no relevance today, particularly in fields where the basic business processes change drastically year-over-year, such as mobile eCommerce. If you have reams of unstructured and disjointed data, you may have too much “cleaning” to do before you can ever get around to learning from the information collected.
UBER’s Head of Machine Learning, Danny Lange, once recommended that companies just starting out in machine learning should begin by applying supervised machine learning to historical data. Find data that’s already clean and relatively recent, and use labelled training data to start finding insights. Note that in a rapidly changing field, newer data is positively required. For example, if you run a door delivery service for pet supplies, and your app, prices, product offerings, and service areas have changed significantly over the last six months, you will need much more recent data to learn from than, say, a company selling homeowners’ insurance in Montana. If data is not related to the relevant trends and nuances of your current business, it is unlikely to glean predictive value.
Key questions for enterprise executives (continued)
Have I thought about the total costs involved?
Lots of data means lots of storage. While the per-gigabyte cost of storage is sharply lower than it used to be, you can still run up a sizable tab. That’s before you’ve even considered computational costs: How much data do you analyse? How often? Who does it? In many cases, a data scientist may be needed to coordinate efforts. A cost analysis of the options is required to help make an informed decision.
Can your solution to this problem afford for some allowance of error?
ML might be thought of as a kind of “skill”, in the same sense that one might apply the word to human beings. A skill that’s alive, adapting, growing and informed by experience. For this reason, an ML solution will often be incorrect a certain percentage of the time, especially when it’s informed by new or varied stimuli. If your task absolutely cannot allow for any error, ML is likely to be the wrong tool for the job.
An example of an application that cannot allow for error might be an application that aims to read the amount of an invoice or bill and then pay that invoice or bill. One letter difference or one number difference could mean overpaying your bill by 10x the original amount (if the decimal was interpreted to be in the wrong place) or sending money to the wrong company (if an invoicing company name isn’t registered exactly).
In a case like above, some degree of ML might help with “bucketing” different types of bills or invoices, but the final decision to enter the payment amount and send a payment would likely require an accountable human.
Conclusion
Rules-based structured data is well on its way to being completely automated. The next phase of intelligent automation has truly arrived. As the majority of the data gathered by enterprises are in an unstructured form, it becomes a necessity to deploy cognitive computing models to take advantage of artificial intelligence. Enterprise intelligent automation has gone cold and needs a layer of machine learning. There are applicable use cases in IoT, NLP and Predictive Analytics from which the enterprise should benefit in the present. Applying cognitive computing is a necessary immediate step to be taken in the evolution of global corporate enterprise.
References
1. Siddiqui, A. (2018, August 7). Distinguishing between cognitive computing and AI. Intelegain.com.
2. Marr, B. (2019, October 16). What Is Unstructured Data And Why Is It So Important To Businesses? An Easy Explanation For Anyone. Forbes. Forbes.com.
3. Matthews, K (2019, April 5), 5 Ways the Travel Industry is Embracing IoT, IoTForAll.com
4. Kassner, M. (2014, April 16). The Internet of Things will force companies to rethink data-center operations. Techrepublic.com.
5. Marr, B. (2017, August 24). Disney Uses Big Data, IoT And Machine Learning To Boost Customer Experience. Forbes. Forbes.com.
6. Your face is your password. (2018, May 15). Hsbc.com.
7. Davis, J. (2019, June 12). NLP, Machine Learning: Killer Combo for Unstructured Data. Information Week. Informationweek.com.
8. Nallagatla, D. (2019, June 3). Using Text Analytics and NLP: An Introduction. Transforming Data With Intelligence. Twdi.org.
9. Groenfeldt, T. (2016, Jun 27) Citi Uses Voice Prints Forbes
10. Griffiths, M. (2017, Mar 20) Citi Tops 1 Million Mark Citi
11. Bobriakov, I (2019, Dec 21) Top NLP Algorithms Data Science Central
12. Chatbot: What is a Chatbot? Why are Chatbots Important? (2018, July 17). Expertsystem.com.
13. Targett, E. (2019, December 12). “Erica” Hits 10 Million Users. Computer Business Review. Cbronline.com.
14. Macheel, T. (2017, July 28). Inside the development of Erica, Bank of America’s AI-powered bot. Tearsheet. Tearsheet.co.
15. Buchanan, J. (2018, May 8). Buoy Health, CVS Partner on Chatbot That Helps Patients Find Care. Xconomy. Xconomy.com.
16. Sunajro, E. (2019, September 23). How Travel Brands are Using Conversational AI. Icons8.com.
17. Iulia, C. (2019, September 25). How AI transformed Amazon into an eCommerce powerhouse. Brandminds.ro.
18. Hanifin, B. (2019, October 3). What Artificial Intelligence Means For Customer Loyalty Marketing. Forbes.
19. Some, K. (2018, August 10). Top Challenges Business Enterprises Face In Implementing Artificial Intelligence Solutions. Analytics Insight. Analyticinsight. net.
20. How to Use Machine Learning to Solve Business Problems. (2018, June 14). Softmedialab.com.
21. Faggella, D. (2018, November 29). UBER’s Head of Machine Learning Thinks You Might be Doing it Wrong. Emerj.com.